Bandwidth & Second Level Digital Divide Post COVID
EDUA 6381 – Critical Issue in Ed Tech
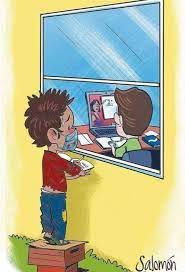
Education during the COVID-19 pandemic underwent significant changes. Schools had to shift from in-person teaching to online learning instruction. But what about students who did not have access to the necessary digital tools, equipment, and internet at home to continue with their education?
As mentioned in the video “The 'Digital Divide' and COVID-19's Impact on Internet Access,” (2021) the term Digital Divide is “the gap between those with computer and internet access and those without.” The video also highlighted how COVID-19 affected students differently. For instance, there was one student who had no access to the internet and had to go to parking lots in search of a Wi-Fi signal to participate in remote learning. It is particularly difficult for low-income minority households, primarily Latino and Black, to access the internet due to a lack of suitable devices. internet connectivity.
As communicated in “The 'Digital Divide' and COVID-19's Impact on Internet Access” (2021), internet companies have used old technology to provide low-speed internet service to minority communities. The pandemic has brought attention to all these issues and highlighted the significant challenge that our country must solve.
Although government programs like Emergency Broadband Benefit program are available, minority neighborhoods' quality of internet, speed (download and upload speeds), and latency impact students' ability to have quality connections to download materials and even more important now, uploading has become even more demanding.
Education utilizes data daily through different systems, including learning management, student information, enterprise resource planning, data warehouses, longitudinal data systems, vendor systems, and countless devices. As mentioned in the Predictive Analytics article from SREB (2015), “Education leaders can also use predictive models based on descriptive data and diagnostics to choose educational technology systems that would better serve their goals for educational improvement.”
In the article “Predictive Modeling of K-12 Academic Outcomes,” Porter and Balu stated that predictive modeling in education could assist educators “to identify students who are at risk of not meeting key academic outcomes, such as graduating on time or passing state exams.” The authors believe that it is important that teachers identify early warning systems. Data is collected from all different sources and compiled into these predictive modeling sets that schools and teachers utilize to basically profile students’ risks from attaining success.
Porter and Balu highlighted that “the use of ABC indicator approach, indicating that student with low attendance rate, behavior incidents, or course failures may be designated as having a moderate or high risk of not graduating at the end of twelfth grade, depending on how the student’s first-semester outcomes compare with district specified threshold.” Predictive models can be useful as an educator. However, it is crucial to conduct thorough research on both the successes and failures of these models.
As an educator, handling data with care and caution is crucial. Although predictive models can be useful, they might be errors in the data due to inconsistencies in the quality and quantity of information entered into predictive models. Students are individuals whose capabilities to succeed many times rest on their commitment and other unknown factors to schools, and stereotyping them into predetermined groups may have a detrimental impact.
How many students get identified and grouped incorrectly, and what their impact has on their goal to graduate and move on to higher learning? I would like to know more about this and other tools and ensure we are just not letting raw data determine the way we are teaching our students and forget that good old fashion interaction and getting to know them on a personal level, many times reveal more about their character than any statistical model.
For future reference:
Predictive Analytics in K-12: Advantages, Limitations & Implementation By Vasuki Rethinam
References
Mashable. (2021, April 26). The “Digital Divide” and COVID-19’s impact on internet access | Mashable [Video]. YouTube. https://www.youtube.com/watch?v=xkbZPAJF88k
Porter, K. E., Balu, R. (2023, March 28). Predictive modeling of K-12 academic outcomes. MDRC. https://www.mdrc.org/publication/predictive-modeling-k-12-academic-outcomes
Southern Regional Education Board (2015). Predictive Analytics – Southern Regional Education Board. [Review of 10 Issues in Educational Technology]. Southern Regional Education Board. https://www.sreb.org/predictiveanalytics
Kommentare